Conversation starters and points of orientation
Pulling back the curtain
The release of the currently free to use chatbot ChatGPT in late November 2022 put the capabilities of large language models (LLMs) into the hands of mere mortals. GPT-3 from which ChatGPT was built has been available since June 2020 for a small charge. The parent Large Language Model or LLM, GTP-3 has a better capacity to process text than the chatbot but lacks the chatbot’s conversational interface.
Chattiness makes the difference. For formal education, the release of ChatGPT put AI firmly on the education agenda; it’s the noisy canary in the AI coal mine. When its capabilities to write credible undergraduate essays or pass medical exams was noticed the predictable reaction—let’s ban it’s use—played out in many formal education institutions worldwide. In this moment, a Janus-like reaction to ChatGPT became evident. Bans looked to the past: “our graduates don’t/won’t/can’t cheat”. Uses looked to the future: “our graduates are well prepared for an AI-infused future.”
This is a familiar tension. Over the history of the use of digital developments in formal education the playbook has remained the same: ban it (good luck) or domesticate it: make it fit within your existing world. Neither response plays out as imagined but typically they tend to play out over longish time periods. This time it is different. The rate of take up of ChatGPT is the fastest in take up of any digital technology. And it this that has left formal education systems scrambling to work out what to do.
In preparing this piece we often felt we were also scrambling to keep abreast of things as reports of usage, reactions, commentary and more and more AI-based apps appeared rapidly and, at the time of writing, show no signs of slowing down. To put it more bluntly, we felt like wall-paper hangers, nursing babies while decorating their nursery in the midst of a tropical Queensland cyclone.
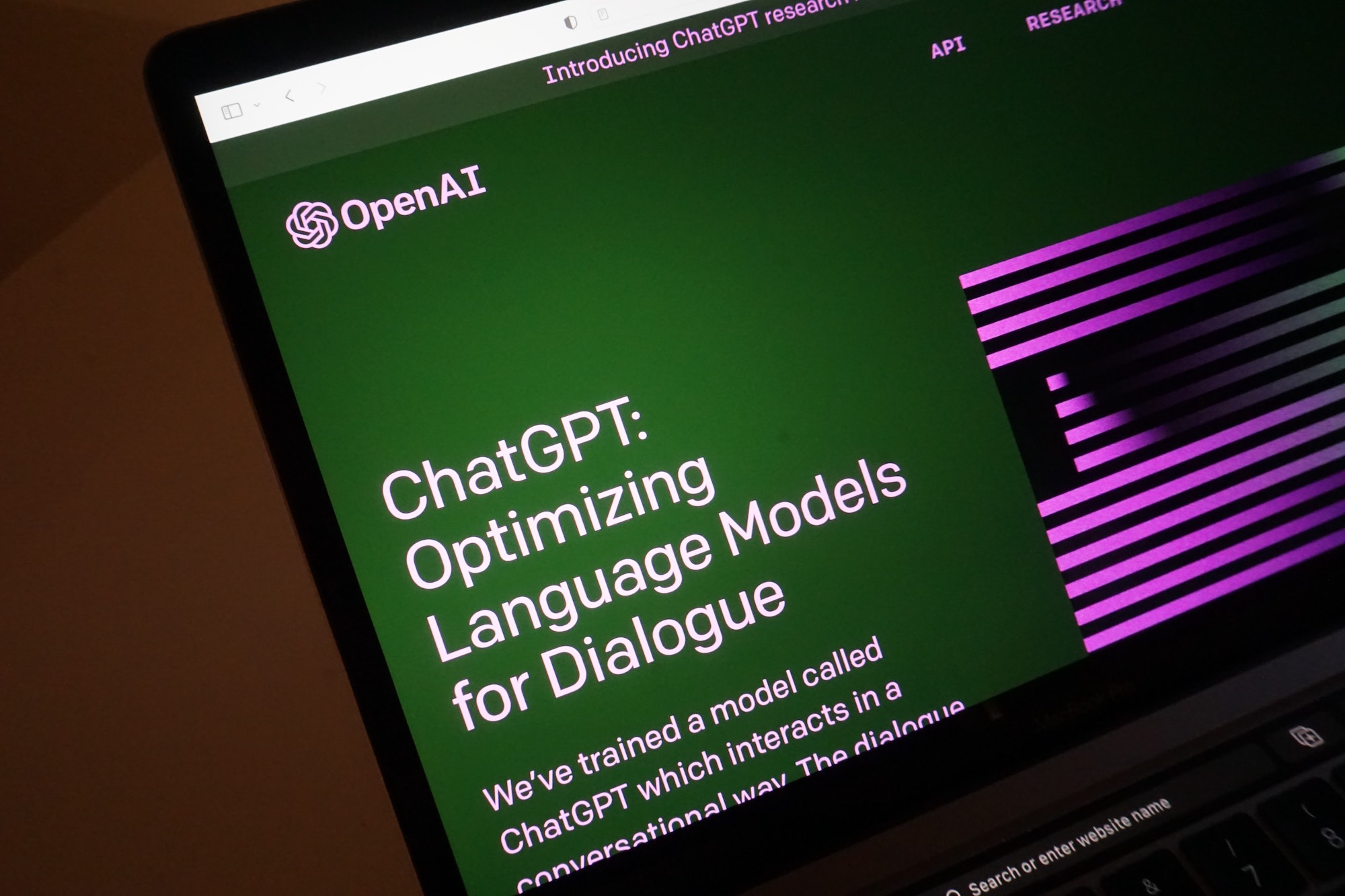
Stepping back to take a breath
We think it is useful to point out that while the release of GPT-3 and then ChatGPT appeared to be sudden, it masked the many years of slow developments in AI of which we have had only odd glimpses. Reports of a machine beating a world champion chess or Go player and, more interestingly, developments in medical diagnosis, protein folding, the law, music and so on.
As some commentary likes to describe it, all that has happened with the release of LLMs is that the curtain has been pulled back on them. It is only one curtain though and it takes attention away from still largely veiled to the public AI developments. As Yann LeCunn, Chief AI scientist at meta put it ”On the highway towards Human-Level AI, Large Language Model is an off-ramp.”
It’s impossible in this short piece to do justice to any kind of mapping of other developments in AI. All that might be observed is that investment in AI research, application and development is huge and likely to keep us busy for a long time.
Given all of that, a key question remains for educators and ChatGPT.
What to do on Monday?
The cover of Douglas Adam’s A Hitchhiker’s Guide to the Galaxy is good advice: “Don’t Panic”. But don’t be smug either.
Whenever a new technology appears it is commonly understood in terms of analogies with what is familiar and well known. ChatGPT has been commonly described as a conversational Google search or a conversational database. This assumption leads us to a confusing space. After a Google search we have to select or curate our ‘answers’ assuming at least some responsibility for accuracy. At times ChatGPT provides useful, even accurate information but it also can and does provide output that is politely described as hallucinatory. It will give answers that are not correct. It will provide references to papers that do not exist. It will (if pressed) change its ‘mind’. It is not a shortcut to ‘the truth’.
The confusion arises because of a poor or limited understanding of how the model was built and how it operates. We believe that a fundamental first step, before Monday, is to develop a rough working understanding of the model which can inform how we work with.
GPT-3 was trained on a large amount of text that was available online. It processes the text in small chunks (called tokens) and establishes numerical patterns of association between them. When the model is prompted, it acts like the autocomplete on your phone. It predicts the next word in a sequence based on the context provided by the previous word – a bit like that co-worker who always wants to try and finish your sentences.
So ChatGPT is a chatbot that outputs fluent, plausible text that reflects the body of text that was online up to the middle of 2021. This leads to experiences which reflect the wide range of views, biases and prejudices of English-speaking people (who are probably, also, able-bodied, middle class, cis-gendered and white). (Ask ChatGPT for an opinion on Shakespeare and you’ll get the idea). To address the problem of producing toxic text, OpenAI, the company that produced the GPT series of LLMs—outsourced the work of identifying either incorrect responses or a text that a subscribed found unhelpful/inadequate/offensive to a company that employed Kenyan workers on very low wages. These people manually tag or label large volumes of text snippets drawn from the “darkest recesses of the Internet”. The manually tagged text snippets were then used to fine tune the model.
The fine tuning of the model continues via the interactions of users since the release of ChatGPT. The text shown prior to use of the bot indicates, “Conversations may be reviewed by our AI trainers to improve our systems”. You can experiment with the bot by finding a fact that is wrong and correcting it. The correction may result in a minor tweaking that will give a more accurate response in a subsequent conversation with it but not necessarily.
Back to Monday
With a rough idea of how it works, to understand and get a good relationship with ChatGPT you need to use it. A lot. You specifically need to spend a lot of time playing with prompts. What counts in any interaction with ChatGPT is the quality of your questions or prompts. The more detail and context you can provide in a prompt the more useful (to you) the output from the bot is likely to be. Asking for an example of a haiku will produce one kind of response. Asking for a gender-inclusive haiku that reflects the style of Buffy the Vampire Slayer will give you something else. There are many examples online of “creative prompting” or prompt wrangling. As with any new way of doing things you have to use it, play with it, explore what it can and can’t do as well as what it will and won’t do.
If prompt wrangling feels like work, we can take comfort from the fact every educator on the planet with access to ChatGPT is working through this challenge right now. This is a good thing. These are our people. When responding to fast moving innovations like AI we can try and fly solo but a far more productive approach is to work with colleagues, peers: those nearby and those far away; those we already know, and those we have yet to meet.
“For students from culturally and linguistically diverse backgrounds who routinely report challenges with communication as barriers to their education, this might be a game changer.”
How to negotiate the use of ChatGPT with students?
What ChatGPT does well is produce what some have described as beige text, which is unsurprising given the corpus it was trained on. So, things like university mission statements, lesson plans, outlines of research reports, job descriptions, press releases, lists of key performance indicators, policy documents, assessment questions, rubrics, assignment descriptions and so on are easily generated. So too are summaries of text and counter arguments to a text.
When it comes to educational practices, it would be naive to assume students won’t make use of such a resource. Equally naive (or, in fact, just offensive) is the assumption that most students will use it to cheat. How to negotiate the use of ChatGPT with students is fundamentally an educational problem, not a technical one. There are all manner of opportunities here, too many to list in a short piece. An important equity opportunity is that for students whose written English is seen as poor, the bot can provide a useful first draft of an essay or report, or identify and explain an error in a sentence, or decipher and explain as many times as needed a tutor’s feedback on a draft. The student can engage the bot in conversation, a one-to-one tutor if you like, to explain an idea, an argument, the use of a phrase. Unlike a tutor, or colleague or study friend, the bot doesn’t get tired. It doesn’t mind how many times you ask a question; it has an enormous repertoire of analogies or metaphors to draw upon, and can increase or decrease the difficulty of an explanation on request. This is not support that University’s seem able to offer from tutors.
For students from culturally and linguistically diverse backgrounds who routinely report challenges with communication as barriers to their education, this might be a game changer. On the other hand, of course, we risk losing the individuality inherent in people’s more natural expression. And that would definitely be a loss.
We did ask ChatGPT to improve this text. We stuck with what we wrote. This points to the third of what we think of as complementary skills for using GPT-3, an ability to judge the quality and accuracy of the output. ChatGPT could say a lot more to us, but for now, the big challenge is for us to be curious, rather than frightened, and to look carefully at the claims (both positive and negative) that are made about our new AI. There was so much we wanted to say. We hope this is a useful conversation starter.
Authors
Professor Chris Bigum is an Adjunct Professor in the Griffith Institute for Educational Research at Griffith University.
Professor Leonie Rowan is the Director of the Griffith Institute for Educational Research at Griffith University.
Professor Rowan’s research and teaching interests focus on three key inter-related areas: gender and education, University teaching and learning Educational and social justice. Her research and teaching are fundamentally interconnected and build on more than two decades of experience in education settings. She has received numerous, prestigious awards for her teaching and multiple competitive research grants (including 6 ARC funded grants) which signal the quality and impact of her work.
Share
Share
Share